v1.0 of presentation.
|
@ -2,3 +2,8 @@
|
|||
*.swp
|
||||
__pycache__
|
||||
tmp.py
|
||||
.env
|
||||
*.aux
|
||||
*.log
|
||||
*.out
|
||||
tmp.*
|
||||
|
|
|
@ -0,0 +1,11 @@
|
|||
.PHONY:to_wwu
|
||||
|
||||
all: to_wwu
|
||||
|
||||
to_wwu:
|
||||
rsync -avz ~/577/repo/docs/figures/ linux-04:/home/jensen33/Dev/studentweb/assets/static/577/
|
||||
scp ~/577/repo/docs/presentation.md linux-04:/home/jensen33/Dev/studentweb/content/577/contents.lr
|
||||
scp ~/Dev/www.publicmatt.com/models/slides.ini linux-04:/home/jensen33/Dev/studentweb/models/
|
||||
scp ~/Dev/www.publicmatt.com/templates/slides.html linux-04:/home/jensen33/Dev/studentweb/templates/
|
||||
rsync -avz ~/Dev/www.publicmatt.com/assets/static/revealjs linux-04:/home/jensen33/Dev/studentweb/assets/static/
|
||||
ssh linux-04 cd /home/jensen33/Dev/studentweb \; make
|
|
@ -0,0 +1,3 @@
|
|||
paper.pdf: paper.tex
|
||||
pdflatex $^ -o $@
|
||||
evince $@
|
After Width: | Height: | Size: 47 KiB |
After Width: | Height: | Size: 22 KiB |
After Width: | Height: | Size: 24 KiB |
After Width: | Height: | Size: 20 KiB |
After Width: | Height: | Size: 33 KiB |
After Width: | Height: | Size: 29 KiB |
After Width: | Height: | Size: 48 KiB |
After Width: | Height: | Size: 61 KiB |
After Width: | Height: | Size: 51 KiB |
After Width: | Height: | Size: 22 KiB |
After Width: | Height: | Size: 54 KiB |
|
@ -0,0 +1,61 @@
|
|||
\documentclass{article}
|
||||
\usepackage{multicol}
|
||||
\usepackage{hyperref}
|
||||
\title{Data Mining CS 571}
|
||||
\author{Matt Jensen}
|
||||
\date{2023-04-25}
|
||||
|
||||
\begin{document}
|
||||
\maketitle
|
||||
|
||||
\section*{Abstract}
|
||||
|
||||
News organizations have been repeatedly accused of being partisan.
|
||||
Additionally, they have been accused of polarizing dicussion to drive up revenue and engagement.
|
||||
This paper seeks to quantify those claims by classifying the degree to which news headlines have become more emotionally charged of time.
|
||||
A secondary goal is the investigate whether news organization have been uniformly polarized, or if one pole has been 'moving' more rapidly away from the 'middle'.
|
||||
This analysis will probe to what degree has the \href{https://en.wikipedia.org/wiki/Overton_window}{Overton Window} has shifted in the media.
|
||||
Naom Chomsky had a hypothesis about manufactured consent that is beyond the scope of this paper, so we will restrict our analysis to the presence of agenda instead of the cause of it.
|
||||
|
||||
\begin{multicols}{2}
|
||||
|
||||
\section{Data Preparation}
|
||||
The subject of analysis is a set of news article headlines scraped from the news aggregation site \href{https://mememorandum.com}{Memeorandum} for news stories from 2006 to 2022.
|
||||
Each news article has a title, author, description, publisher, publish date, url and related discussions.
|
||||
The site also has a concept of references, where a main, popular story may be covered by other sources.
|
||||
This link association might be used to support one or more of the hypothesis of the main analysis.
|
||||
After scraping the site, the data will need to be deduplicated and normalized to minimize storage costs and processing errors.
|
||||
What remains after these cleaning steps is approximitely 6,400 days of material, 300,000 distinct headlines from 21,000 publishers and 34,000 authors used in the study.
|
||||
|
||||
\section{Missing Data Policy}
|
||||
|
||||
The largest data policy that will have to be dealt with is news organizations that share the same parent company, but might have slightly different names.
|
||||
Wall Street Journal news is drastically different than their opinion section.
|
||||
Other organizations have slightly different names for the same thing and a product of the aggregation service and not due to any real difference.
|
||||
Luckily, most of the anaylsis is operating on the content of the news headlines, which do not suffer from this data impurity.
|
||||
|
||||
\section{Classification Task}
|
||||
|
||||
The classification of news titles into emotional categories was accomplished by using a pretrained large langauge model from \href{https://huggingface.co/arpanghoshal/EmoRoBERTa}{HuggingFace}.
|
||||
This model was trained on \href{https://ai.googleblog.com/2021/10/goemotions-dataset-for-fine-grained.html}{a dataset curated and published by Google} which manually classified a collection of 58,000 comments into 28 emotions.
|
||||
The classes for each article will be derived by tokenizing the title and running the model over the tokens, then grabbing the largest probabilty class from the output.
|
||||
|
||||
The data has been discretized into years.
|
||||
Additionally, the publishers will have been discretized based of either principle component analysis on link similarity or based on the bias ratings of \href{https://www.allsides.com/media-bias/ratings}{All Sides}.
|
||||
Given that the features of the dataset are sparse, it is not expected to have any useless attributes, unless the original hypothesis of a temporal trend proving to be false.
|
||||
Of the features used in the analysis, there are enough data points that null or missing values can safely be excluded.
|
||||
|
||||
\section{Experiments}
|
||||
|
||||
No computational experiment have been done yet.
|
||||
Generating the tokenized text, the word embedding and the emotional sentiment analysis have made up the bulk of the work thus far.
|
||||
The bias ratings do not cover all publisher in the dataset, so the number of articles without a bias rating from their publisher will have to be calculated.
|
||||
If it is less than 30\% of the articles, it might not make sense to use the bias ratings.
|
||||
The creation and reduction of the link graph with principle component analysis will need to be done to visualize the relationship between related publishers.
|
||||
|
||||
\section{Results}
|
||||
\textbf{TODO.}
|
||||
|
||||
\end{multicols}
|
||||
|
||||
\end{document}
|
|
@ -0,0 +1,552 @@
|
|||
_model: slides
|
||||
---
|
||||
|
||||
title: CSCI 577 - Data Mining
|
||||
|
||||
---
|
||||
body:
|
||||
|
||||
# Political Polarization
|
||||
|
||||
Matt Jensen
|
||||
|
||||
===
|
||||
|
||||
# Hypothesis
|
||||
|
||||
Political polarization is rising, and news articles are a proxy measure.
|
||||
|
||||
==
|
||||
|
||||
# Is this reasonable?
|
||||
|
||||
|
||||
==
|
||||
|
||||
# Why is polarization rising?
|
||||
|
||||
Not my job, but there's research<sup>[ref](#references)</sup> to support it
|
||||
|
||||
|
||||
==
|
||||
|
||||
# Sub-hypothesis
|
||||
|
||||
- The polarization increases near elections. <!-- .element: class="fragment" -->
|
||||
- The polarization is not evenly distributed across publishers. <!-- .element: class="fragment" -->
|
||||
- The polarization is not evenly distributed across political specturm. <!-- .element: class="fragment" -->
|
||||
|
||||
==
|
||||
|
||||
# Sub-sub-hypothesis
|
||||
|
||||
- Similarly polarized publishers link to each other. <!-- .element: class="fragment" -->
|
||||
- 'Mainstream' media uses more neutral titles. <!-- .element: class="fragment" -->
|
||||
- Highly polarized publications don't last as long. <!-- .element: class="fragment" -->
|
||||
|
||||
===
|
||||
|
||||
# Data Source(s)
|
||||
|
||||
memeorandum.com <!-- .element: class="fragment" -->
|
||||
|
||||
allsides.com <!-- .element: class="fragment" -->
|
||||
|
||||
huggingface.com <!-- .element: class="fragment" -->
|
||||
|
||||
===
|
||||
|
||||
<section data-background-iframe="https://www.memeorandum.com" data-background-interactive></section>
|
||||
|
||||
===
|
||||
|
||||
# memeorandum.com
|
||||
|
||||
- News aggregation site. <!-- .element: class="fragment" -->
|
||||
- Was really famous before Google News. <!-- .element: class="fragment" -->
|
||||
- Still aggregates sites today. <!-- .element: class="fragment" -->
|
||||
|
||||
==
|
||||
|
||||
# Why Memeorandum?
|
||||
|
||||
- Behavioral: I only read titles sometimes. (doom scrolling). <!-- .element class="fragment" -->
|
||||
- Behavioral: It's my source of news (with sister site TechMeme.com). <!-- .element class="fragment" -->
|
||||
- Convenient: most publishers block bots. <!-- .element class="fragment" -->
|
||||
- Convenient: dead simple html to parse. <!-- .element class="fragment" -->
|
||||
- Archival: all headlines from 2006 forward. <!-- .element class="fragment" -->
|
||||
- Archival: automated, not editorialized. <!-- .element class="fragment" -->
|
||||
|
||||
===
|
||||
|
||||
<section data-background-iframe="https://www.allsides.com/media-bias/ratings" data-background-interactive></section>
|
||||
|
||||
===
|
||||
|
||||
# AllSides.com
|
||||
|
||||
- Rates news publications as left, center or right. <!-- .element: class="fragment" -->
|
||||
- Ratings combine: <!-- .element: class="fragment" -->
|
||||
- blind bias surveys.
|
||||
- editorial reviews.
|
||||
- third party research.
|
||||
- community voting.
|
||||
- Originally scraped website, but direct access eventually. <!-- .element: class="fragment" -->
|
||||
|
||||
|
||||
==
|
||||
|
||||
# Why AllSides?
|
||||
|
||||
- Behavioral: One of the first google results on bias apis. <!-- .element class="fragment" -->
|
||||
- Convenient: Ordinal ratings [-2: very left, 2: very right]. <!-- .element class="fragment" -->
|
||||
- Convenient: Easy format. <!-- .element class="fragment" -->
|
||||
- Archival: Covers 1400 publishers. <!-- .element class="fragment" -->
|
||||
|
||||
===
|
||||
|
||||
<section data-background-iframe="https://huggingface.co/models" data-background-interactive></section>
|
||||
|
||||
===
|
||||
|
||||
# HuggingFace.com
|
||||
|
||||
- Deep Learning library. <!-- .element: class="fragment" -->
|
||||
- Lots of pretrained models. <!-- .element: class="fragment" -->
|
||||
- Easy, off the shelf word/sentence embeddings and text classification models. <!-- .element: class="fragment" -->
|
||||
|
||||
==
|
||||
|
||||
# Why HuggingFace?
|
||||
|
||||
- Behavioral: Language Models are HOT right now. <!-- .element: class="fragment" -->
|
||||
- Behavioral: The dataset needed more features.<!-- .element: class="fragment" -->
|
||||
- Convenient: Literally 5 lines of python.<!-- .element: class="fragment" -->
|
||||
- Convenient: Testing different model performance was easy.<!-- .element: class="fragment" -->
|
||||
- Archival: Lots of pretrained classification tasks.<!-- .element: class="fragment" -->
|
||||
|
||||
===
|
||||
|
||||
# Data Structures
|
||||
Stories
|
||||
|
||||
- Top level stories. <!-- .element: class="fragment" -->
|
||||
- title.
|
||||
- publisher.
|
||||
- author.
|
||||
- Related discussion. <!-- .element: class="fragment" -->
|
||||
- publisher.
|
||||
- uses 'parent' story as a source.
|
||||
- Stream of stories (changes constantly). <!-- .element: class="fragment" -->
|
||||
|
||||
==
|
||||
|
||||
# Data Structures
|
||||
Bias
|
||||
|
||||
- Per publisher. <!-- .element: class="fragment" -->
|
||||
- name.
|
||||
- label.
|
||||
- agree/disagree vote by community.
|
||||
- Name could be semi-automatically joined to stories. <!-- .element: class="fragment" -->
|
||||
|
||||
==
|
||||
|
||||
# Data Structures
|
||||
Embeddings
|
||||
|
||||
- Per story title. <!-- .element: class="fragment" -->
|
||||
- sentence embedding (n, 384).
|
||||
- sentiment classification (n, 1).
|
||||
- emotional classification (n, 1).
|
||||
- ~ 1 hour of inference time to map story titles and descriptions. <!-- .element: class="fragment" -->
|
||||
|
||||
===
|
||||
|
||||
# Data Collection
|
||||
|
||||
==
|
||||
|
||||
# Data Collection
|
||||
|
||||
Story Scraper (simplified)
|
||||
|
||||
```python
|
||||
day = timedelta(days=1)
|
||||
cur = date(2005, 10, 1)
|
||||
end = date.today()
|
||||
while cur <= end:
|
||||
cur = cur + day
|
||||
save_as = output_dir / f"{cur.strftime('%y-%m-%d')}.html"
|
||||
url = f"https://www.memeorandum.com/{cur.strftime('%y%m%d')}/h2000"
|
||||
r = requests.get(url)
|
||||
with open(save_as, 'w') as f:
|
||||
f.write(r.text)
|
||||
```
|
||||
|
||||
==
|
||||
|
||||
# Data Collection
|
||||
Bias Scraper (hard)
|
||||
|
||||
```python
|
||||
...
|
||||
bias_html = DATA_DIR / 'allsides.html'
|
||||
parser = etree.HTMLParser()
|
||||
tree = etree.parse(str(bias_html), parser)
|
||||
root = tree.getroot()
|
||||
rows = root.xpath('//table[contains(@class,"views-table")]/tbody/tr')
|
||||
|
||||
ratings = []
|
||||
for row in rows:
|
||||
rating = dict()
|
||||
...
|
||||
```
|
||||
|
||||
==
|
||||
|
||||
# Data Collection
|
||||
Bias Scraper (easy)
|
||||
|
||||
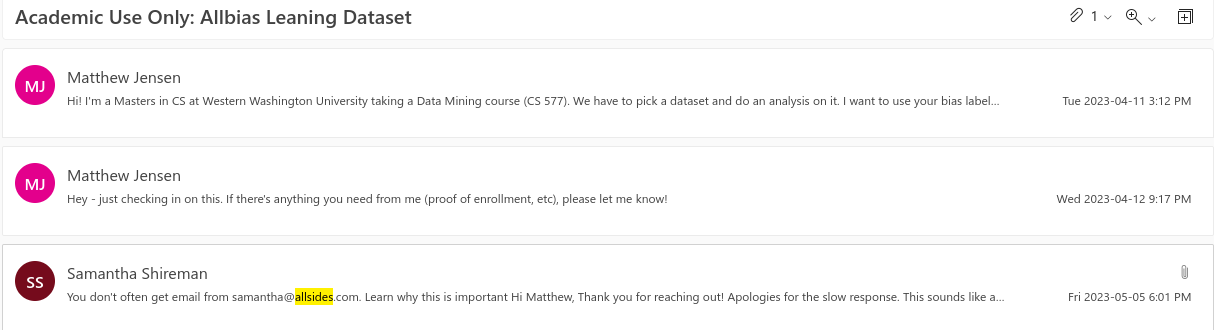
|
||||
|
||||
==
|
||||
|
||||
# Data Collection
|
||||
Embeddings (easy)
|
||||
|
||||
```python
|
||||
# table = ...
|
||||
tokenizer = AutoTokenizer.from_pretrained("roberta-base")
|
||||
model = AutoModel.from_pretrained("roberta-base")
|
||||
|
||||
for chunk in table:
|
||||
tokens = tokenizer(chunk, add_special_tokens = True, truncation = True, padding = "max_length", max_length=92, return_attention_mask = True, return_tensors = "pt")
|
||||
outputs = model(**tokens)
|
||||
embeddings = outputs.last_hidden_state.detach().numpy()
|
||||
...
|
||||
```
|
||||
|
||||
==
|
||||
|
||||
# Data Collection
|
||||
Classification Embeddings (medium)
|
||||
|
||||
```python
|
||||
...
|
||||
outputs = model(**tokens)[0].detach().numpy()
|
||||
scores = 1 / (1 + np.exp(-outputs)) # Sigmoid
|
||||
class_ids = np.argmax(scores, axis=1)
|
||||
for i, class_id in enumerate(class_ids):
|
||||
results.append({"story_id": ids[i], "label" : model.config.id2label[class_id]})
|
||||
...
|
||||
```
|
||||
|
||||
===
|
||||
|
||||
# Data Selection
|
||||
|
||||
==
|
||||
|
||||
# Data Selection
|
||||
Stories
|
||||
|
||||
- Clip the first and last full year of stories. <!-- .element: class="fragment" -->
|
||||
- Remove duplicate stories (big stories span multiple days). <!-- .element: class="fragment" -->
|
||||
|
||||
==
|
||||
# Data Selection
|
||||
|
||||
Publishers
|
||||
|
||||
- Combine subdomains of stories. <!-- .element: class="fragment" -->
|
||||
- blog.washingtonpost.com and washingtonpost.com are considered the same publisher.
|
||||
- This could be bad. For example: opinion.wsj.com != wsj.com.
|
||||
|
||||
==
|
||||
|
||||
# Data Selection
|
||||
|
||||
Links
|
||||
|
||||
- Select only stories with publishers whose story had been a 'parent' ('original publishers'). <!-- .element: class="fragment" -->
|
||||
- Eliminates small blogs and non-original news.
|
||||
- Eliminate publishers without links to original publishers. <!-- .element: class="fragment" -->
|
||||
- Eliminate silo'ed publications.
|
||||
- Link matrix is square and low'ish dimensional.
|
||||
|
||||
==
|
||||
|
||||
# Data Selection
|
||||
|
||||
Bias
|
||||
|
||||
- Keep all ratings, even ones with low agree/disagree ratio.
|
||||
- Join datasets on publisher name.
|
||||
- Not automatic (look up Named Entity Recognition). <!-- .element: class="fragment" -->
|
||||
- Started with 'jaro winkler similarity' then manually from there.
|
||||
- Use numeric values
|
||||
- [left: -2, left-center: -1, ...]
|
||||
|
||||
===
|
||||
|
||||
# Descriptive Stats
|
||||
|
||||
Raw
|
||||
|
||||
| metric | value |
|
||||
|:------------------|--------:|
|
||||
| total stories | 299714 |
|
||||
| total related | 960111 |
|
||||
| publishers | 7031 |
|
||||
| authors | 34346 |
|
||||
| max year | 2023 |
|
||||
| min year | 2005 |
|
||||
| top level domains | 7063 |
|
||||
|
||||
==
|
||||
# Descriptive Stats
|
||||
|
||||
Stories Per Publisher
|
||||
|
||||

|
||||
|
||||
==
|
||||
|
||||
# Descriptive Stats
|
||||
|
||||
Top Publishers
|
||||
|
||||
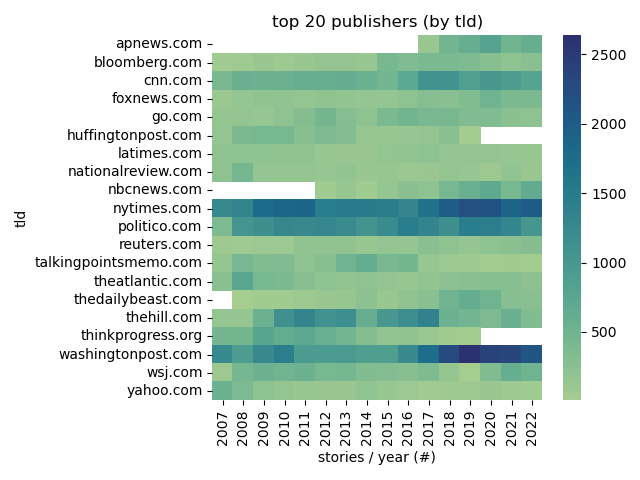
|
||||
|
||||
==
|
||||
|
||||
# Descriptive Stats
|
||||
|
||||
Articles Per Year
|
||||
|
||||
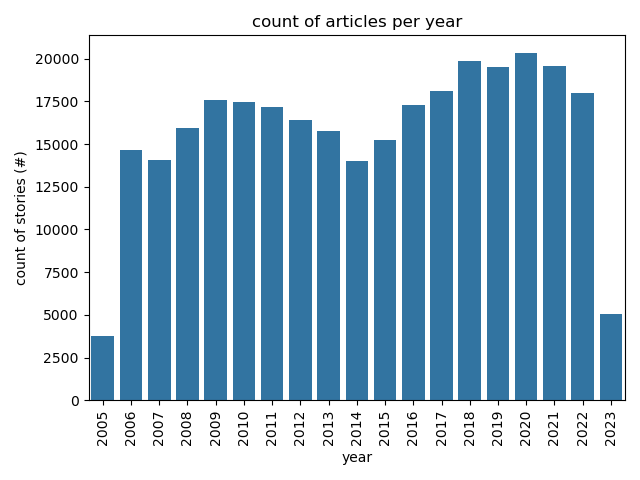
|
||||
|
||||
==
|
||||
|
||||
# Descriptive Stats
|
||||
|
||||
Common TLDs
|
||||
|
||||
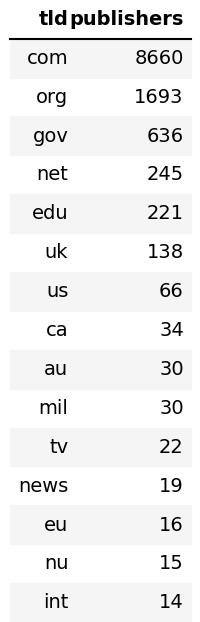
|
||||
|
||||
==
|
||||
|
||||
# Descriptive Stats
|
||||
|
||||
Post Process
|
||||
|
||||
| key | value |
|
||||
|:------------------|--------:|
|
||||
| total stories | 251553 |
|
||||
| total related | 815183 |
|
||||
| publishers | 223 |
|
||||
| authors | 23809 |
|
||||
| max year | 2022 |
|
||||
| min year | 2006 |
|
||||
| top level domains | 234 |
|
||||
|
||||
===
|
||||
# Experiments
|
||||
|
||||
1. **clustering** on link similarity. <!-- .element: class="fragment" -->
|
||||
2. **classification** on link similarity. <!-- .element: class="fragment" -->
|
||||
3. **classification** on sentence embedding. <!-- .element: class="fragment" -->
|
||||
4. **classification** on sentiment analysis. <!-- .element: class="fragment" -->
|
||||
5. **regression** on emotional classification over time and publication. <!-- .element: class="fragment" -->
|
||||
|
||||
===
|
||||
# Experiment 1
|
||||
|
||||
Setup
|
||||
|
||||
- Create one-hot encoding of links between publishers. <!-- .element: class="fragment" -->
|
||||
- Cluster the encoding. <!-- .element: class="fragment" -->
|
||||
- Expect similar publications in same cluster. <!-- .element: class="fragment" -->
|
||||
- Use PCA to visualize clusters. <!-- .element: class="fragment" -->
|
||||
|
||||
Note:
|
||||
Principle Component Analysis:
|
||||
- a statistical technique for reducing the dimensionality of a dataset.
|
||||
- linear transformation into a new coordinate system where (most of) the variation data can be described with fewer dimensions than the initial data.
|
||||
|
||||
==
|
||||
|
||||
# Experiment 1
|
||||
|
||||
One Hot Encoding
|
||||
|
||||
| publisher | nytimes| wsj| newsweek| ...|
|
||||
|:----------|--------:|----:|--------:|----:|
|
||||
| nytimes | 1| 1| 1| ...|
|
||||
| wsj | 1| 1| 0| ...|
|
||||
| newsweek | 0| 0| 1| ...|
|
||||
| ... | ...| ...| ...| ...|
|
||||
|
||||
==
|
||||
|
||||
# Experiment 1
|
||||
|
||||
n-Hot Encoding
|
||||
|
||||
| publisher | nytimes| wsj| newsweek| ...|
|
||||
|:----------|--------:|----:|--------:|----:|
|
||||
| nytimes | 11| 1| 141| ...|
|
||||
| wsj | 1| 31| 0| ...|
|
||||
| newsweek | 0| 0| 1| ...|
|
||||
| ... | ...| ...| ...| ...|
|
||||
|
||||
==
|
||||
|
||||
# Experiment 1
|
||||
|
||||
Normalized n-Hot Encoding
|
||||
|
||||
| publisher | nytimes| wsj| newsweek| ...|
|
||||
|:----------|--------:|----:|--------:|----:|
|
||||
| nytimes | 0| 0.4| 0.2| ...|
|
||||
| wsj | 0.2| 0| 0.4| ...|
|
||||
| newsweek | 0.0| 0.0| 0.0| ...|
|
||||
| ... | ...| ...| ...| ...|
|
||||
|
||||
==
|
||||
|
||||
# Experiment 1
|
||||
|
||||
Elbow criterion
|
||||
|
||||
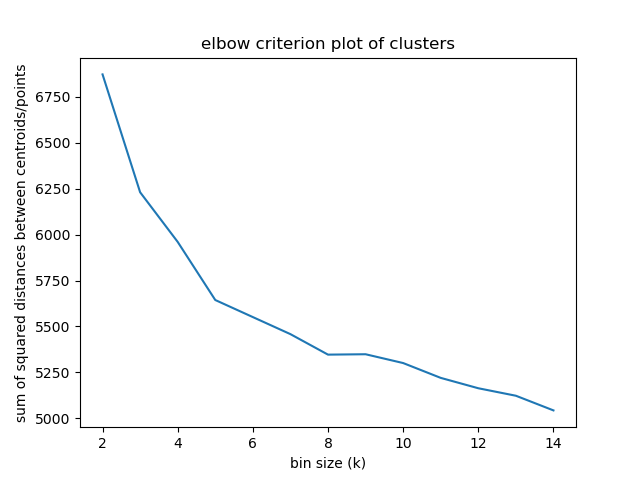
|
||||
|
||||
Note:
|
||||
|
||||
The elbow method looks at the percentage of explained variance as a function of the number of clusters:
|
||||
|
||||
One should choose a number of clusters so that adding another cluster doesn't give much better modeling of the data.
|
||||
|
||||
Percentage of variance explained is the ratio of the between-group variance to the total variance,
|
||||
|
||||
==
|
||||
|
||||
# Experiment 1
|
||||
|
||||
Link Magnitude
|
||||
|
||||
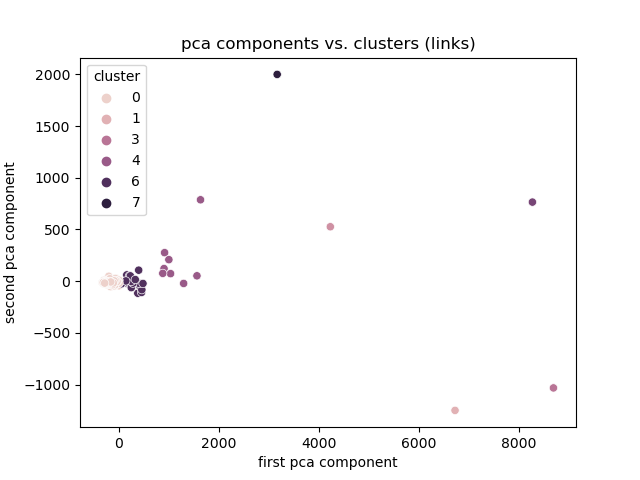
|
||||
|
||||
==
|
||||
|
||||
# Experiment 1
|
||||
|
||||
Normalized
|
||||
|
||||
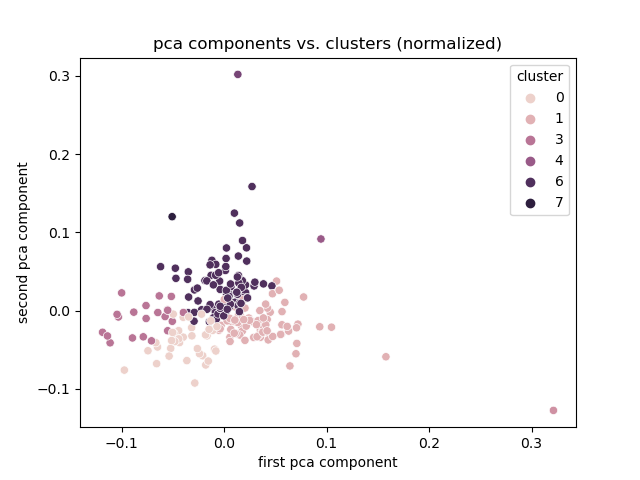
|
||||
|
||||
==
|
||||
|
||||
# Experiment 1
|
||||
|
||||
Onehot
|
||||
|
||||
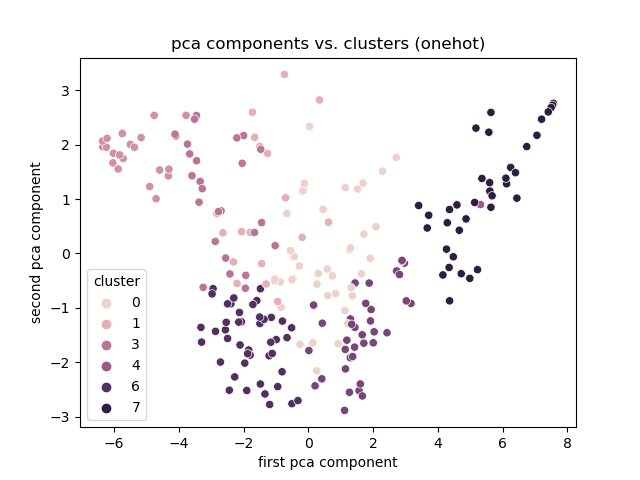
|
||||
|
||||
==
|
||||
|
||||
# Experiment 1
|
||||
|
||||
Discussion
|
||||
|
||||
- Best encoding: One hot. <!-- .element: class="fragment" -->
|
||||
- Clusters based on total links otherwise.
|
||||
- Clusters, but no explanation
|
||||
- Limitation: need the link encoding to cluster.
|
||||
- Smaller publishers might not link very much.
|
||||
|
||||
===
|
||||
|
||||
# Experiment 2
|
||||
|
||||
Setup
|
||||
|
||||
- Create features. <!-- .element: class="fragment" -->:
|
||||
- Publisher frequency.
|
||||
- Reuse link encodings.
|
||||
- Create classes: <!-- .element: class="fragment" -->
|
||||
- Join bias classifications.
|
||||
- Train classifier. <!-- .element: class="fragment" -->
|
||||
|
||||
Note:
|
||||
|
||||
==
|
||||
# Experiment 2
|
||||
Descriptive stats
|
||||
|
||||
| metric | value |
|
||||
|:------------|:----------|
|
||||
| publishers | 1582 |
|
||||
| labels | 6 |
|
||||
| left | 482 |
|
||||
| center | 711 |
|
||||
| right | 369 |
|
||||
| agree range | [0.0-1.0] |
|
||||
|
||||
==
|
||||
|
||||
# Experiment 2
|
||||
|
||||
PCA + Labels
|
||||
|
||||
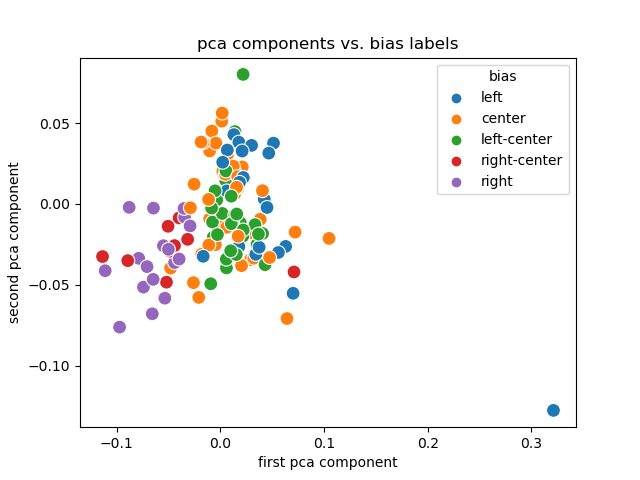
|
||||
|
||||
==
|
||||
|
||||
# Experiment 2
|
||||
|
||||
Discussion
|
||||
|
||||
- Link encodings (and their PCA) are useful. <!-- .element: class="fragment" -->
|
||||
- Labels are (sort of) separated and clustered.
|
||||
- Creating them for smaller publishers is trivial.
|
||||
==
|
||||
|
||||
# Experiment 2
|
||||
|
||||
Limitations
|
||||
|
||||
- Dependent on accurate rating. <!-- .element: class="fragment" -->
|
||||
- Ordinal ratings not available. <!-- .element: class="fragment" -->
|
||||
- Dependent on accurate joining across datasets. <!-- .element: class="fragment" -->
|
||||
- Entire publication is rated, not authors. <!-- .element: class="fragment" -->
|
||||
- Don't know what to do with community rating. <!-- .element: class="fragment" -->
|
||||
|
||||
===
|
||||
|
||||
# Experiment 3
|
||||
|
||||
Setup
|
||||
|
||||
==
|
||||
|
||||
# Limitations
|
||||
|
||||
- Many different authors under the same publisher. <!-- .element: class="fragment" -->
|
||||
- Publishers use syndication. <!-- .element: class="fragment" -->
|
||||
- Bias ratings are biased. <!-- .element: class="fragment" -->
|
||||
|
||||
===
|
||||
|
||||
# Questions
|
||||
|
||||
===
|
||||
|
||||
<!-- .section: id="references" -->
|
||||
|
||||
# References
|
||||
|
||||
[1]: Stewart, A.J. et al. 2020. Polarization under rising inequality and economic decline. Science Advances. 6, 50 (Dec. 2020), eabd4201. DOI:https://doi.org/10.1126/sciadv.abd4201.
|
||||
|
||||
Note:
|
218
src/bias.py
|
@ -1,12 +1,12 @@
|
|||
import click
|
||||
from data import connect
|
||||
from data.main import connect
|
||||
import pandas as pd
|
||||
from lxml import etree
|
||||
from pathlib import Path
|
||||
import os
|
||||
import csv
|
||||
|
||||
def map(rating:str) -> int:
|
||||
def label_to_int(rating:str) -> int:
|
||||
|
||||
mapping = {
|
||||
'left' : 0,
|
||||
|
@ -19,20 +19,18 @@ def map(rating:str) -> int:
|
|||
|
||||
return mapping[rating]
|
||||
|
||||
def int_to_label(class_id: int) -> str:
|
||||
mapping = {
|
||||
0 : 'left',
|
||||
1 : 'left-center',
|
||||
2 : 'center',
|
||||
3 : 'right-center',
|
||||
4 : 'right',
|
||||
-1 : 'allsides',
|
||||
}
|
||||
return mapping[class_id]
|
||||
|
||||
@click.command(name="bias:load")
|
||||
def load() -> None:
|
||||
DB = connect()
|
||||
DATA_DIR = Path(os.environ['DATA_MINING_DATA_DIR'])
|
||||
f = str(DATA_DIR / "bias_ratings.csv")
|
||||
|
||||
DB.sql(f"""
|
||||
create table bias_ratings as
|
||||
select
|
||||
row_number() over(order by b.publisher) as id
|
||||
,b.*
|
||||
from read_csv_auto('{f}') b
|
||||
""")
|
||||
@click.command(name="bias:normalize")
|
||||
def normalize() -> None:
|
||||
DB = connect()
|
||||
|
@ -41,133 +39,48 @@ def normalize() -> None:
|
|||
CREATE OR REPLACE TABLE publisher_bias AS
|
||||
WITH cte AS (
|
||||
SELECT
|
||||
p.id
|
||||
p.id as publisher_id
|
||||
,b.id as bias_id
|
||||
,b.bias as label
|
||||
,JARO_WINKLER_SIMILARITY(LOWER(p.name), LOWER(b.publisher)) as similarity
|
||||
FROM bias_ratings b
|
||||
JOIN publishers p
|
||||
JOIN top.publishers p
|
||||
ON JARO_WINKLER_SIMILARITY(LOWER(p.name), LOWER(b.publisher)) > 0.95
|
||||
),ranked AS (
|
||||
SELECT
|
||||
id
|
||||
publisher_id
|
||||
,bias_id
|
||||
,label
|
||||
,similarity
|
||||
,ROW_NUMBER() OVER(PARTITION BY id ORDER BY similarity DESC) AS rn
|
||||
,ROW_NUMBER() OVER(PARTITION BY publisher_id ORDER BY similarity DESC) AS rn
|
||||
FROM cte
|
||||
)
|
||||
SELECT
|
||||
id
|
||||
publisher_id
|
||||
,label
|
||||
,bias_id
|
||||
FROM ranked
|
||||
WHERE ranked.rn = 1
|
||||
""")
|
||||
|
||||
mapping = [
|
||||
{'label' :'left' , 'ordinal': -2},
|
||||
{'label' :'left-center' , 'ordinal': -1},
|
||||
{'label' :'center' , 'ordinal': 0},
|
||||
{'label' :'right-center' , 'ordinal': 1},
|
||||
{'label' :'right' , 'ordinal': 2},
|
||||
]
|
||||
mapping = pd.DataFrame(mapping)
|
||||
|
||||
DB.sql("""
|
||||
with cte as (
|
||||
select
|
||||
s.publisher_id
|
||||
,count(1) as stories
|
||||
from stories s
|
||||
group by s.publisher_id
|
||||
)
|
||||
select
|
||||
s.publisher
|
||||
,s.stories
|
||||
,b.publisher
|
||||
,b.bias
|
||||
from bias_ratings b
|
||||
join cte s
|
||||
on s.publisher = b.publisher
|
||||
order by
|
||||
stories desc
|
||||
limit 15
|
||||
DB.query("alter table bias_ratings add column ordinal int")
|
||||
|
||||
DB.query("""
|
||||
update bias_ratings b
|
||||
set ordinal = o.ordinal
|
||||
FROM mapping o
|
||||
WHERE o.label = b.bias
|
||||
""")
|
||||
|
||||
DB.sql("""
|
||||
with cte as (
|
||||
select
|
||||
s.publisher
|
||||
,count(1) as stories
|
||||
from stories s
|
||||
group by s.publisher
|
||||
)
|
||||
select
|
||||
sum(stories)
|
||||
,avg(agree / disagree)
|
||||
from bias_ratings b
|
||||
join cte s
|
||||
on s.publisher = b.publisher
|
||||
""")
|
||||
|
||||
DB.sql("""
|
||||
with cte as (
|
||||
select
|
||||
s.publisher
|
||||
,count(1) as stories
|
||||
from stories s
|
||||
group by s.publisher
|
||||
)
|
||||
select
|
||||
sum(s.stories) filter(where b.publisher is not null) as matched
|
||||
,sum(s.stories) filter(where b.publisher is null) as unmatched
|
||||
,cast(sum(s.stories) filter(where b.publisher is not null) as numeric)
|
||||
/ sum(s.stories) filter(where b.publisher is null) as precent_matched
|
||||
from bias_ratings b
|
||||
right join cte s
|
||||
on s.publisher = b.publisher
|
||||
""")
|
||||
|
||||
DB.sql("""
|
||||
select
|
||||
*
|
||||
from bias_ratings
|
||||
where publisher ilike '%CNN%'
|
||||
""")
|
||||
|
||||
@click.command(name='bias:debug')
|
||||
def debug() -> None:
|
||||
DB = connect()
|
||||
DATA_DIR = Path(os.environ['DATA_MINING_DATA_DIR'])
|
||||
f = str(DATA_DIR / "bias_ratings.csv")
|
||||
|
||||
DB.sql("""
|
||||
with cte as (
|
||||
select
|
||||
outlet
|
||||
,count(1) as stories
|
||||
from stories
|
||||
group by outlet
|
||||
)
|
||||
,total as (
|
||||
select
|
||||
sum(stories) as total
|
||||
from cte
|
||||
)
|
||||
select
|
||||
cte.outlet
|
||||
,cte.stories
|
||||
,bias.outlet
|
||||
,bias.lean
|
||||
,sum(100 * (cte.stories / cast(total.total as float))) over() as rep
|
||||
,total.total
|
||||
from cte
|
||||
join bias
|
||||
on jaro_winkler_similarity(bias.outlet, cte.outlet) > 0.9
|
||||
cross join total.total
|
||||
""")
|
||||
|
||||
DB.sql("""
|
||||
select
|
||||
outlet
|
||||
,count(1) as stories
|
||||
from stories
|
||||
group by outlet
|
||||
order by count(1) desc
|
||||
limit 50
|
||||
""")
|
||||
|
||||
outlets
|
||||
|
||||
@click.command(name='bias:parse')
|
||||
def parse() -> None:
|
||||
|
@ -199,3 +112,64 @@ def parse() -> None:
|
|||
ratings.append(rating)
|
||||
df = pd.DataFrame(ratings)
|
||||
df.to_csv(DATA_DIR / 'bias_ratings.csv', sep="|", index=False, quoting=csv.QUOTE_NONNUMERIC)
|
||||
|
||||
@click.command(name="bias:load")
|
||||
def load() -> None:
|
||||
DB = connect()
|
||||
DATA_DIR = Path(os.environ['DATA_MINING_DATA_DIR'])
|
||||
f = str(DATA_DIR / "bias_ratings.csv")
|
||||
|
||||
DB.sql(f"""
|
||||
CREATE TABLE bias_ratings as
|
||||
select
|
||||
row_number() over(order by b.publisher) as id
|
||||
,b.*
|
||||
from read_csv_auto('{f}') b
|
||||
""")
|
||||
|
||||
@click.command('bias:export')
|
||||
def export():
|
||||
data_path = Path(os.environ['DATA_MINING_DATA_DIR'])
|
||||
|
||||
DB = connect()
|
||||
all_bias = DB.query("""
|
||||
SELECT
|
||||
id as bias_id
|
||||
,publisher as name
|
||||
,bias as label
|
||||
FROM bias_ratings
|
||||
ORDER by agree desc
|
||||
""")
|
||||
all_bias.df().to_csv(data_path / 'TMP_publisher_bias.csv', sep="|", index=False)
|
||||
mapped_bias = DB.query("""
|
||||
SELECT
|
||||
p.id as publisher_id
|
||||
,p.name as name
|
||||
,p.tld as tld
|
||||
,b.label as bias
|
||||
,b.bias_id as bias_id
|
||||
FROM top.publishers p
|
||||
LEFT JOIN publisher_bias b
|
||||
ON b.publisher_id = p.id
|
||||
""")
|
||||
mapped_bias.df().to_csv(data_path / 'TMP_publisher_bias_to_load.csv', sep="|", index=False)
|
||||
DB.close()
|
||||
|
||||
@click.command('bias:import-mapped')
|
||||
def import_mapped():
|
||||
data_path = Path(os.environ['DATA_MINING_DATA_DIR'])
|
||||
table_name = "top.publisher_bias"
|
||||
|
||||
DB = connect()
|
||||
df = pd.read_csv(data_path / 'TMP_publisher_bias_to_load.csv', sep="|")
|
||||
|
||||
DB.query(f"""
|
||||
CREATE OR REPLACE TABLE {table_name} AS
|
||||
SELECT
|
||||
publisher_id AS publisher_id
|
||||
,cast(bias_id AS int) as bias_id
|
||||
FROM df
|
||||
WHERE bias_id IS NOT NULL
|
||||
""")
|
||||
print(f"created table: {table_name}")
|
||||
|
||||
|
|
24
src/cli.py
|
@ -7,7 +7,7 @@ def cli():
|
|||
|
||||
if __name__ == "__main__":
|
||||
load_dotenv()
|
||||
import scrape
|
||||
from data import scrape
|
||||
cli.add_command(scrape.download)
|
||||
cli.add_command(scrape.parse)
|
||||
cli.add_command(scrape.load)
|
||||
|
@ -32,4 +32,26 @@ if __name__ == "__main__":
|
|||
cli.add_command(emotion.create_table)
|
||||
import sentence
|
||||
cli.add_command(sentence.embed)
|
||||
from train import main as train_main
|
||||
cli.add_command(train_main.main)
|
||||
|
||||
import plots.descriptive as plotd
|
||||
cli.add_command(plotd.articles_per_year)
|
||||
cli.add_command(plotd.distinct_publishers)
|
||||
cli.add_command(plotd.stories_per_publisher)
|
||||
cli.add_command(plotd.top_publishers)
|
||||
cli.add_command(plotd.common_tld)
|
||||
|
||||
import links as linkcli
|
||||
cli.add_command(linkcli.create_table)
|
||||
cli.add_command(linkcli.create_pca)
|
||||
cli.add_command(linkcli.create_clusters)
|
||||
|
||||
import plots.links as plotl
|
||||
cli.add_command(plotl.elbow)
|
||||
cli.add_command(plotl.link_pca_clusters)
|
||||
|
||||
import plots.classifier as plotc
|
||||
cli.add_command(plotc.pca_with_classes)
|
||||
|
||||
cli()
|
||||
|
|
|
@ -0,0 +1,6 @@
|
|||
import data.main
|
||||
import data.scrape
|
||||
__all__ = [
|
||||
'main'
|
||||
,'scrape'
|
||||
]
|
|
@ -4,10 +4,12 @@ import requests
|
|||
from pathlib import Path
|
||||
import click
|
||||
from tqdm import tqdm
|
||||
from data import data_dir, connect
|
||||
from data.main import data_dir, connect
|
||||
from lxml import etree
|
||||
import pandas as pd
|
||||
from urllib.parse import urlparse
|
||||
from tld import get_tld
|
||||
from tld.utils import update_tld_names
|
||||
|
||||
@click.command(name='scrape:load')
|
||||
@click.option('--directory', type=Path, default=data_dir(), show_default=True)
|
||||
|
@ -61,6 +63,7 @@ def download(output_dir):
|
|||
@click.option('-o', '--output_dir', type=Path, default=data_dir(), show_default=True)
|
||||
def parse(directory, output_dir):
|
||||
"""parse the html files on disk into a structured csv format."""
|
||||
update_tld_names()
|
||||
directory = data_dir() / "memeorandum"
|
||||
parser = etree.HTMLParser()
|
||||
pages = [f for f in directory.glob("*.html")]
|
||||
|
@ -104,8 +107,7 @@ def parse(directory, output_dir):
|
|||
|
||||
url = item.xpath('.//strong/a')[0].get('href')
|
||||
out['url'] = url
|
||||
out['publisher_url_domain'] = urlparse(publisher_url).netloc
|
||||
out['domain'] = urlparse(url).netloc
|
||||
out['tld'] = get_tld(publisher_url)
|
||||
|
||||
item_id = hash((page.stem, url))
|
||||
out['id'] = item_id
|
||||
|
@ -225,3 +227,111 @@ def normalize():
|
|||
alter table related_stories drop publisher_domain;
|
||||
""")
|
||||
|
||||
|
||||
def another_norm():
|
||||
sv2 = pd.read_csv(data_dir / 'stories.csv', sep="|")
|
||||
related = pd.read_csv(data_dir / 'related.csv', sep="|")
|
||||
|
||||
related['tld'] = related.url.apply(lambda x: map_tld(x))
|
||||
|
||||
DB.query("""
|
||||
update related_stories
|
||||
set publisher_id = p.id
|
||||
from publishers p
|
||||
join related r
|
||||
on r.tld = p.tld
|
||||
where r.url = related_stories.url
|
||||
""")
|
||||
|
||||
|
||||
DB.query("""alter table stories add column tld text""")
|
||||
|
||||
s_url = DB.query("""
|
||||
select
|
||||
id
|
||||
,url
|
||||
from stories
|
||||
""").df()
|
||||
|
||||
|
||||
s_url['tld'] = s_url.url.apply(lambda x: map_tld(x))
|
||||
|
||||
DB.query("""
|
||||
update stories
|
||||
set tld = s_url.tld
|
||||
from s_url
|
||||
where s_url.id = stories.id
|
||||
""")
|
||||
|
||||
DB.query("""
|
||||
update stories
|
||||
set publisher_id = p.id
|
||||
from publishers p
|
||||
where p.tld = stories.tld
|
||||
""")
|
||||
|
||||
|
||||
select
|
||||
DB.query("""
|
||||
update stories
|
||||
set stories.publisher_id = p.id
|
||||
from new_pub
|
||||
""")
|
||||
sv2['tld'] = sv2.publisher_url.apply(lambda x: map_tld(x))
|
||||
|
||||
|
||||
new_pub = DB.query("""
|
||||
with cte as (
|
||||
select
|
||||
tld
|
||||
,publisher
|
||||
,count(1) filter(where year(published_at) = 2022) as recent_ctn
|
||||
,count(1) as ctn
|
||||
from sv2
|
||||
group by
|
||||
tld
|
||||
,publisher
|
||||
)
|
||||
,r as (
|
||||
select
|
||||
tld
|
||||
,publisher
|
||||
,ctn
|
||||
,row_number() over(partition by tld order by recent_ctn desc) as rn
|
||||
from cte
|
||||
)
|
||||
select
|
||||
row_number() over() as id
|
||||
,publisher as name
|
||||
,tld
|
||||
from r
|
||||
where rn = 1
|
||||
order by ctn desc
|
||||
""").df()
|
||||
|
||||
DB.query("""
|
||||
CREATE OR REPLACE TABLE publishers AS
|
||||
SELECT
|
||||
id
|
||||
,name
|
||||
,tld
|
||||
FROM new_pub
|
||||
""")
|
||||
|
||||
|
||||
def map_tld(x):
|
||||
try:
|
||||
res = get_tld(x, as_object=True)
|
||||
return res.fld
|
||||
except:
|
||||
return None
|
||||
|
||||
DB.sql("""
|
||||
SELECT
|
||||
s.id
|
||||
,sv2.publisher_url
|
||||
FROM stories s
|
||||
JOIN sv2
|
||||
on sv2.id = s.id
|
||||
limit 5
|
||||
""")
|
|
@ -6,7 +6,7 @@ import numpy as np
|
|||
|
||||
from transformers import BertTokenizer
|
||||
from model import BertForMultiLabelClassification
|
||||
from data import connect, data_dir
|
||||
from data.main import connect, data_dir
|
||||
import seaborn as sns
|
||||
import matplotlib.pyplot as plt
|
||||
from matplotlib.dates import DateFormatter
|
||||
|
@ -376,3 +376,99 @@ def debug():
|
|||
DB.close()
|
||||
|
||||
out.to_csv(data_dir() / 'emotions.csv', sep="|")
|
||||
|
||||
def another():
|
||||
DB = connect()
|
||||
DB.sql("""
|
||||
select
|
||||
*
|
||||
from emotions
|
||||
""")
|
||||
|
||||
emotions = DB.sql("""
|
||||
select
|
||||
year(s.published_at) as year
|
||||
,se.label as emotion
|
||||
,count(1) as stories
|
||||
from stories s
|
||||
join story_emotions se
|
||||
on s.id = se.story_id
|
||||
group by
|
||||
year(s.published_at)
|
||||
,se.label
|
||||
""").df()
|
||||
|
||||
sns.scatterplot(x=emotions['year'], y=emotions['stories'], hue=emotions['emotion'])
|
||||
plt.show()
|
||||
|
||||
pivot = emotions.pivot(index='year', columns='emotion', values='stories')
|
||||
pivot.reset_index(inplace=True)
|
||||
from sklearn.linear_model import LinearRegression
|
||||
reg = LinearRegression()
|
||||
|
||||
for emotion in pivot.keys()[1:].tolist():
|
||||
_ = reg.fit(pivot['year'].to_numpy().reshape(-1, 1), pivot[emotion])
|
||||
print(f"{emotion}: {reg.coef_[0]}")
|
||||
|
||||
fig, ax = plt.subplots()
|
||||
#sns.lineplot(x=pivot['anger'], y=pivot['joy'])
|
||||
#sns.lineplot(x=pivot['anger'], y=pivot['surprise'], ax=ax)
|
||||
sns.lineplot(x=pivot['anger'], y=pivot['fear'], ax=ax)
|
||||
sns.lineplot(x=pivot[''], y=pivot['fear'], ax=ax)
|
||||
plt.show()
|
||||
|
||||
DB.close()
|
||||
|
||||
normalized = DB.sql("""
|
||||
with cte as (
|
||||
select
|
||||
year(s.published_at) as year
|
||||
,se.label as emotion
|
||||
,b.label as bias
|
||||
from stories s
|
||||
join story_emotions se
|
||||
on s.id = se.story_id
|
||||
join publisher_bias b
|
||||
on b.id = s.publisher_id
|
||||
where b.label != 'allsides'
|
||||
and se.label != 'neutral'
|
||||
)
|
||||
select
|
||||
distinct
|
||||
year
|
||||
,emotion
|
||||
,bias
|
||||
,cast(count(1) over(partition by year, bias, emotion) as float) / count(1) over(partition by year, bias) as group_count
|
||||
from cte
|
||||
""").df()
|
||||
|
||||
DB.sql("""
|
||||
select
|
||||
b.label as bias
|
||||
,count(1) as stories
|
||||
from stories s
|
||||
join story_emotions se
|
||||
on s.id = se.story_id
|
||||
join publisher_bias b
|
||||
on b.id = s.publisher_id
|
||||
group by
|
||||
b.label
|
||||
""").df()
|
||||
|
||||
another_pivot = emotional_bias.pivot(index=['bias', 'year'], columns='emotion', values='stories')
|
||||
another_pivot.reset_index(inplace=True)
|
||||
|
||||
sns.lineplot(data=normalized, x='year', y='group_count', hue='bias', style='emotion')
|
||||
plt.show()
|
||||
|
||||
sns.relplot(
|
||||
data=normalized, x="year", y="group_count", hue="emotion", col='bias', kind="line"
|
||||
#data=normalized, x="year", y="group_count", hue="emotion", col='bias', kind="line", facet_kws=dict(sharey=False)
|
||||
)
|
||||
plt.show()
|
||||
|
||||
DB.sql("""
|
||||
select
|
||||
*
|
||||
from another_pivot
|
||||
""")
|
||||
|
|
|
@ -1,8 +0,0 @@
|
|||
import sklearn
|
||||
import polars as pl
|
||||
import toml
|
||||
from pathlib import Path
|
||||
|
||||
config = toml.load('/home/user/577/repo/config.toml')
|
||||
app_dir = Path(config.get('app').get('path'))
|
||||
df = pl.read_csv(app_dir / "data/articles.csv")
|
158
src/links.py
|
@ -1,12 +1,148 @@
|
|||
from data import connect
|
||||
import click
|
||||
from data.main import connect
|
||||
import pandas as pd
|
||||
import numpy as np
|
||||
from sklearn.decomposition import PCA, TruncatedSVD
|
||||
from sklearn.cluster import MiniBatchKMeans
|
||||
import seaborn as sns
|
||||
import matplotlib.pyplot as plt
|
||||
|
||||
|
||||
@click.command('links:create-table')
|
||||
def create_table():
|
||||
|
||||
table_name = "top.link_edges"
|
||||
DB = connect()
|
||||
DB.query(f"""
|
||||
CREATE OR REPLACE TABLE {table_name} AS
|
||||
with cte as(
|
||||
SELECT
|
||||
s.publisher_id as parent_id
|
||||
,r.publisher_id as child_id
|
||||
,count(1) as links
|
||||
FROM top.stories s
|
||||
JOIN top.related_stories r
|
||||
ON s.id = r.parent_id
|
||||
group by
|
||||
s.publisher_id
|
||||
,r.publisher_id
|
||||
)
|
||||
SELECT
|
||||
cte.parent_id
|
||||
,cte.child_id
|
||||
,cte.links as links
|
||||
,cast(cte.links as float) / sum(cte.links) over(partition by cte.parent_id) as normalized
|
||||
,case when cte.links > 0 then 1 else 0 end as onehot
|
||||
FROM cte
|
||||
WHERE cte.child_id in (
|
||||
SELECT
|
||||
distinct parent_id
|
||||
FROM cte
|
||||
)
|
||||
AND cte.parent_id in (
|
||||
SELECT
|
||||
distinct child_id
|
||||
FROM cte
|
||||
)
|
||||
""")
|
||||
DB.close()
|
||||
|
||||
DB = connect()
|
||||
DB.query("""
|
||||
SELECT
|
||||
*
|
||||
,-log10(links)
|
||||
--distinct parent_id
|
||||
FROM top.link_edges e
|
||||
WHERE e.parent_id = 238
|
||||
""")
|
||||
DB.close()
|
||||
print(f"created {table_name}")
|
||||
|
||||
@click.command('links:create-pca')
|
||||
@click.option('--source', type=click.Choice(['links', 'normalized', 'onehot']), default='links')
|
||||
def create_pca(source):
|
||||
"""create 2D pca labels"""
|
||||
|
||||
from sklearn.decomposition import PCA
|
||||
|
||||
table_name = f"top.publisher_pca_{source}"
|
||||
DB = connect()
|
||||
pub = DB.query("""
|
||||
SELECT
|
||||
*
|
||||
FROM top.publishers
|
||||
""").df()
|
||||
df = DB.query(f"""
|
||||
SELECT
|
||||
parent_id
|
||||
,child_id
|
||||
,{source} as links
|
||||
FROM top.link_edges
|
||||
""").df()
|
||||
DB.close()
|
||||
pivot = df.pivot(index='parent_id', columns='child_id', values='links').fillna(0)
|
||||
|
||||
svd = PCA(n_components=2)
|
||||
svd_out = svd.fit_transform(pivot)
|
||||
|
||||
out = pivot.reset_index()[['parent_id']]
|
||||
out['first'] = svd_out[:, 0]
|
||||
out['second'] = svd_out[:, 1]
|
||||
out = pd.merge(out, pub, left_on='parent_id', right_on='id')
|
||||
|
||||
DB = connect()
|
||||
DB.query(f"""
|
||||
CREATE OR REPLACE TABLE {table_name} AS
|
||||
SELECT
|
||||
out.id as publisher_id
|
||||
,out.first as first
|
||||
,out.second as second
|
||||
FROM out
|
||||
""")
|
||||
DB.close()
|
||||
print(f"created {table_name}")
|
||||
|
||||
|
||||
@click.command('links:create-clusters')
|
||||
@click.option('--source', type=click.Choice(['links', 'normalized', 'onehot']), default='links')
|
||||
def create_clusters(source):
|
||||
from sklearn.cluster import KMeans
|
||||
|
||||
table_name = f"top.publisher_clusters_{source}"
|
||||
DB = connect()
|
||||
df = DB.query(f"""
|
||||
SELECT
|
||||
parent_id
|
||||
,child_id
|
||||
,{source} as links
|
||||
FROM top.link_edges
|
||||
""").df()
|
||||
pub = DB.query("""
|
||||
SELECT
|
||||
*
|
||||
FROM top.publishers
|
||||
""").df()
|
||||
DB.close()
|
||||
pivot = df.pivot(index='parent_id', columns='child_id', values='links').fillna(0)
|
||||
|
||||
|
||||
k = 8
|
||||
kmeans = KMeans(n_clusters=k, n_init="auto")
|
||||
pred = kmeans.fit_predict(pivot)
|
||||
out = pivot.reset_index()[['parent_id']]
|
||||
out['label'] = pred
|
||||
out = pd.merge(out, pub, left_on='parent_id', right_on='id')
|
||||
new_table = out[['id', 'label']]
|
||||
|
||||
DB = connect()
|
||||
DB.query(f"""
|
||||
CREATE OR REPLACE TABLE {table_name} AS
|
||||
SELECT
|
||||
n.id as publisher_id
|
||||
,n.label as label
|
||||
FROM new_table n
|
||||
""")
|
||||
DB.close()
|
||||
print(f"created {table_name}")
|
||||
|
||||
def to_matrix():
|
||||
"""returns an adjacency matrix of publishers to publisher link frequency"""
|
||||
|
@ -21,6 +157,7 @@ def to_matrix():
|
|||
{'label' :'right', 'value' : 4},
|
||||
{'label' :'allsides', 'value' : -1},
|
||||
])
|
||||
|
||||
bias = DB.sql("""
|
||||
SELECT
|
||||
b.id
|
||||
|
@ -37,11 +174,7 @@ def to_matrix():
|
|||
p.id
|
||||
,p.name
|
||||
,p.url
|
||||
,b.label
|
||||
,b.value
|
||||
from publishers p
|
||||
left join bias b
|
||||
on b.id = p.id
|
||||
""").df()
|
||||
|
||||
edges = DB.sql("""
|
||||
|
@ -81,12 +214,23 @@ def to_matrix():
|
|||
ON p.id = cte.parent_id
|
||||
""").df()
|
||||
|
||||
# only keep values that have more than 1 link
|
||||
test = edges[edges['links'] > 2].pivot(index='parent_id', columns='child_id', values='links').fillna(0).reset_index()
|
||||
edges.dropna().pivot(index='parent_id', columns='child_id', values='links').fillna(0)
|
||||
pd.merge(adj, pub, how='left', left_on='parent_id', right_on='id')
|
||||
adj = edges.pivot(index='parent_id', columns='child_id', values='links').fillna(0)
|
||||
adj.values.shape
|
||||
|
||||
|
||||
out = pd.DataFrame(adj.index.values, columns=['id'])
|
||||
out = pd.merge(out, pub, how='left', on='id')
|
||||
return out
|
||||
|
||||
@click.command('links:analysis')
|
||||
def analysis():
|
||||
from sklearn.decomposition import PCA, TruncatedSVD
|
||||
from sklearn.cluster import MiniBatchKMeans
|
||||
adj = to_matrix()
|
||||
pca = PCA(n_components=4)
|
||||
pca_out = pca.fit_transform(adj)
|
||||
|
||||
|
|
|
@ -1,4 +1,4 @@
|
|||
from data import data_dir, connect
|
||||
from data.main import data_dir, connect
|
||||
import numpy as np
|
||||
import sklearn
|
||||
from sklearn.cluster import MiniBatchKMeans
|
||||
|
|
|
@ -0,0 +1,34 @@
|
|||
import click
|
||||
from data.main import connect
|
||||
import os
|
||||
import seaborn as sns
|
||||
import matplotlib.pyplot as plt
|
||||
from pathlib import Path
|
||||
|
||||
out_dir = Path(os.getenv('DATA_MINING_DOC_DIR')) / 'figures'
|
||||
|
||||
@click.command('plot:pca-with-classes')
|
||||
def pca_with_classes():
|
||||
filename = "pca_with_classes.png"
|
||||
|
||||
DB = connect()
|
||||
data = DB.query(f"""
|
||||
SELECT
|
||||
p.tld
|
||||
,b.bias
|
||||
,c.first
|
||||
,c.second
|
||||
,round(cast(b.agree as float) / (b.agree + b.disagree), 2) ratio
|
||||
FROM top.publishers p
|
||||
JOIN top.publisher_bias pb
|
||||
ON p.id = pb.publisher_id
|
||||
JOIN bias_ratings b
|
||||
ON b.id = pb.bias_id
|
||||
JOIN top.publisher_pca_normalized c
|
||||
ON c.publisher_id = p.id
|
||||
""").df()
|
||||
DB.close()
|
||||
ax = sns.scatterplot(x=data['first'], y=data['second'], hue=data['bias'], s=100)
|
||||
ax.set(title="pca components vs. bias labels", xlabel="first pca component", ylabel="second pca component")
|
||||
plt.savefig(out_dir / filename)
|
||||
print(f"saved: {filename}")
|
|
@ -0,0 +1,302 @@
|
|||
import click
|
||||
from data.main import connect
|
||||
import os
|
||||
import seaborn as sns
|
||||
import matplotlib.pyplot as plt
|
||||
from pathlib import Path
|
||||
import numpy as np
|
||||
|
||||
out_dir = Path(os.getenv('DATA_MINING_DOC_DIR')) / 'figures'
|
||||
|
||||
@click.command('plot:articles-per-year')
|
||||
def articles_per_year():
|
||||
filename = 'articles_per_year.png'
|
||||
|
||||
DB = connect()
|
||||
data = DB.query("""
|
||||
select
|
||||
year(published_at) as year
|
||||
,count(1) as stories
|
||||
from stories
|
||||
group by
|
||||
year(published_at)
|
||||
""").df()
|
||||
DB.close()
|
||||
|
||||
ax = sns.barplot(x=data.year, y=data.stories, color='tab:blue')
|
||||
ax.tick_params(axis='x', rotation=90)
|
||||
ax.set(title="count of articles per year", ylabel="count of stories (#)")
|
||||
plt.tight_layout()
|
||||
plt.savefig(out_dir / filename)
|
||||
|
||||
@click.command('plot:distinct-publishers')
|
||||
def distinct_publishers():
|
||||
filename = 'distinct_publishers.png'
|
||||
|
||||
DB = connect()
|
||||
data = DB.query("""
|
||||
select
|
||||
year(published_at) as year
|
||||
,count(distinct publisher_id) as publishers
|
||||
from stories
|
||||
group by
|
||||
year(published_at)
|
||||
""").df()
|
||||
DB.close()
|
||||
|
||||
ax = sns.barplot(x=data.year, y=data.publishers, color='tab:blue')
|
||||
ax.tick_params(axis='x', rotation=90)
|
||||
ax.set(title="count of publishers per year", ylabel="count of publishers (#)")
|
||||
plt.tight_layout()
|
||||
plt.savefig(out_dir / filename)
|
||||
plt.close()
|
||||
|
||||
@click.command('plot:stories-per-publisher')
|
||||
def stories_per_publisher():
|
||||
filename = 'stories_per_publisher.png'
|
||||
|
||||
DB = connect()
|
||||
data = DB.query("""
|
||||
with cte as (
|
||||
select
|
||||
publisher_id
|
||||
,year(published_at) as year
|
||||
,count(1) as stories
|
||||
from stories
|
||||
group by
|
||||
publisher_id
|
||||
,year(published_at)
|
||||
) , agg as (
|
||||
select
|
||||
publisher_id
|
||||
,avg(stories) as stories_per_year
|
||||
,case
|
||||
when avg(stories) < 2 then 2
|
||||
when avg(stories) < 4 then 4
|
||||
when avg(stories) < 8 then 8
|
||||
when avg(stories) < 16 then 16
|
||||
when avg(stories) < 32 then 32
|
||||
when avg(stories) < 64 then 64
|
||||
when avg(stories) < 128 then 128
|
||||
else 129
|
||||
end as max_avg
|
||||
from cte
|
||||
group by
|
||||
publisher_id
|
||||
)
|
||||
select
|
||||
max_avg
|
||||
,count(1) as publishers
|
||||
from agg
|
||||
group by
|
||||
max_avg
|
||||
""").df()
|
||||
DB.close()
|
||||
|
||||
ax = sns.barplot(x=data.max_avg, y=data.publishers, color='tab:blue')
|
||||
ax.set(title="histogram of publisher stories per year", ylabel="count of publishers (#)", xlabel="max average stories / year")
|
||||
plt.tight_layout()
|
||||
plt.savefig(out_dir / filename)
|
||||
plt.close()
|
||||
|
||||
|
||||
@click.command('plot:top-publishers')
|
||||
def top_publishers():
|
||||
"""plot top publishers over time"""
|
||||
|
||||
filename = 'top_publishers.png'
|
||||
|
||||
DB = connect()
|
||||
data = DB.query("""
|
||||
select
|
||||
p.tld
|
||||
,year(published_at) as year
|
||||
,count(1) as stories
|
||||
from (
|
||||
select
|
||||
p.tld
|
||||
,p.id
|
||||
from top.publishers p
|
||||
join top.stories s
|
||||
on s.publisher_id = p.id
|
||||
group by
|
||||
p.tld
|
||||
,p.id
|
||||
order by count(1) desc
|
||||
limit 20
|
||||
) p
|
||||
join top.stories s
|
||||
on s.publisher_id = p.id
|
||||
group by
|
||||
p.tld
|
||||
,year(published_at)
|
||||
order by count(distinct s.id) desc
|
||||
""").df()
|
||||
DB.close()
|
||||
|
||||
pivot = data.pivot(columns='year', index='tld', values='stories')
|
||||
ax = sns.heatmap(pivot, cmap="crest")
|
||||
ax.set(title="top 20 publishers (by tld)", ylabel="tld", xlabel="stories / year (#)")
|
||||
plt.tight_layout()
|
||||
plt.savefig(out_dir / filename)
|
||||
plt.close()
|
||||
|
||||
|
||||
@click.command('plot:common_tld')
|
||||
def common_tld():
|
||||
import dataframe_image as dfi
|
||||
filename = 'common_tld.png'
|
||||
|
||||
DB = connect()
|
||||
data = DB.query("""
|
||||
select
|
||||
split_part(url, '.', -1) as tld
|
||||
,count(1) as publishers
|
||||
,case when count(1) < 20
|
||||
then string_agg(distinct url, '\t')
|
||||
else NULL
|
||||
end as urls
|
||||
from publishers
|
||||
group by
|
||||
split_part(url, '.', -1)
|
||||
order by
|
||||
count(1) desc
|
||||
""").df()
|
||||
DB.close()
|
||||
data[:15][['tld', 'publishers']].style.hide(axis="index").export_png(out_dir / filename, table_conversion='matplotlib')
|
||||
|
||||
def stats():
|
||||
|
||||
# raw
|
||||
DB.query("""
|
||||
SELECT
|
||||
'total stories' as key
|
||||
,COUNT(1) as value
|
||||
FROM stories
|
||||
UNION
|
||||
SELECT
|
||||
'total related' as key
|
||||
,COUNT(1) as value
|
||||
FROM related_stories
|
||||
UNION
|
||||
SELECT
|
||||
'top level domains' as key
|
||||
,COUNT(distinct tld) as value
|
||||
FROM stories
|
||||
UNION
|
||||
SELECT
|
||||
'publishers' as key
|
||||
,COUNT(1) as value
|
||||
FROM publishers
|
||||
UNION
|
||||
SELECT
|
||||
'authors' as key
|
||||
,COUNT(distinct author) as value
|
||||
FROM stories
|
||||
UNION
|
||||
SELECT
|
||||
'min year' as key
|
||||
,min(year(published_at)) as value
|
||||
FROM stories
|
||||
UNION
|
||||
SELECT
|
||||
'max year' as key
|
||||
,max(year(published_at)) as value
|
||||
FROM stories
|
||||
""").df().to_markdown(index=False)
|
||||
|
||||
# selected
|
||||
DB.query("""
|
||||
SELECT
|
||||
'total stories' as key
|
||||
,COUNT(1) as value
|
||||
FROM top.stories
|
||||
UNION
|
||||
SELECT
|
||||
'total related' as key
|
||||
,COUNT(1) as value
|
||||
FROM top.related_stories
|
||||
UNION
|
||||
SELECT
|
||||
'top level domains' as key
|
||||
,COUNT(distinct tld) as value
|
||||
FROM top.stories
|
||||
UNION
|
||||
SELECT
|
||||
'publishers' as key
|
||||
,COUNT(1) as value
|
||||
FROM top.publishers
|
||||
UNION
|
||||
SELECT
|
||||
'authors' as key
|
||||
,COUNT(distinct author) as value
|
||||
FROM top.stories
|
||||
UNION
|
||||
SELECT
|
||||
'min year' as key
|
||||
,min(year(published_at)) as value
|
||||
FROM top.stories
|
||||
UNION
|
||||
SELECT
|
||||
'max year' as key
|
||||
,max(year(published_at)) as value
|
||||
FROM top.stories
|
||||
""").df().to_markdown(index=False)
|
||||
|
||||
@click.command('plot:bias-stats')
|
||||
def bias_stats():
|
||||
import dataframe_image as dfi
|
||||
filename = 'bias_stats.png'
|
||||
|
||||
DB = connect()
|
||||
|
||||
df = DB.query("""
|
||||
SELECT
|
||||
string_agg(distinct bias)
|
||||
FROM bias_ratings
|
||||
""").df()
|
||||
|
||||
df.keys()
|
||||
|
||||
df = DB.query("""
|
||||
SELECT
|
||||
'publishers' as metric
|
||||
,count(1) as value
|
||||
FROM bias_ratings
|
||||
UNION
|
||||
SELECT
|
||||
'labels' as metric
|
||||
,count(distinct bias) as value
|
||||
FROM bias_ratings
|
||||
UNION
|
||||
SELECT
|
||||
'right' as metric
|
||||
,count(1) as value
|
||||
FROM bias_ratings
|
||||
WHERE bias in ('right', 'right-center')
|
||||
UNION
|
||||
SELECT
|
||||
'left' as metric
|
||||
,count(1) as value
|
||||
FROM bias_ratings
|
||||
WHERE bias in ('left', 'left-center')
|
||||
UNION
|
||||
SELECT
|
||||
'center' as metric
|
||||
,count(1) as value
|
||||
FROM bias_ratings
|
||||
WHERE bias in ('center')
|
||||
UNION
|
||||
SELECT
|
||||
'agree_range' as metric
|
||||
,'['
|
||||
|| min(cast(agree as float) / (agree + disagree))
|
||||
|| '-'
|
||||
|| max(cast(agree as float) / (agree + disagree))
|
||||
|| ']'
|
||||
as value
|
||||
FROM bias_ratings
|
||||
WHERE bias in ('center')
|
||||
""").df()
|
||||
DB.close()
|
||||
print(df.to_markdown(index=False))
|
|
@ -0,0 +1,114 @@
|
|||
import click
|
||||
from data.main import connect
|
||||
from links import to_matrix
|
||||
import os
|
||||
import seaborn as sns
|
||||
import matplotlib.pyplot as plt
|
||||
from pathlib import Path
|
||||
import numpy as np
|
||||
from sklearn.metrics import silhouette_score
|
||||
import pandas as pd
|
||||
|
||||
out_dir = Path(os.getenv('DATA_MINING_DOC_DIR')) / 'figures'
|
||||
|
||||
@click.command('plot:link-elbow')
|
||||
def elbow():
|
||||
from sklearn.cluster import KMeans
|
||||
|
||||
filename = 'link_cluster_elbow.png'
|
||||
|
||||
DB = connect()
|
||||
df = DB.query("""
|
||||
SELECT
|
||||
*
|
||||
FROM link_edges
|
||||
""").df()
|
||||
pivot = df.pivot(index='parent_id', columns='child_id', values='links').fillna(0)
|
||||
|
||||
to_plot = []
|
||||
for k in range(2, 15):
|
||||
kmeans = KMeans(n_clusters=k, n_init="auto")
|
||||
kmeans.fit(pivot)
|
||||
label = kmeans.labels_
|
||||
coeff = silhouette_score(pivot, label, metric='euclidean')
|
||||
to_plot.append({'k': k, 'inertia' : kmeans.inertia_, 'coeff': coeff})
|
||||
to_plot = pd.DataFrame(to_plot)
|
||||
|
||||
ax = sns.lineplot(x=to_plot.k, y=to_plot.inertia)
|
||||
ax.set(title="elbow criterion plot of clusters", xlabel="bin size (k)", ylabel="sum of squared distances between centroids/points")
|
||||
plt.savefig(out_dir / filename)
|
||||
plt.close()
|
||||
|
||||
# randomly pick 8
|
||||
|
||||
@click.command('plot:link-pca-clusters')
|
||||
@click.option('--source', type=click.Choice(['links', 'normalized', 'onehot']), default='links')
|
||||
def link_pca_clusters(source):
|
||||
|
||||
filename = f"link_pca_clusters_{source}.png"
|
||||
|
||||
DB = connect()
|
||||
df = DB.query(f"""
|
||||
SELECT
|
||||
c.label as cluster
|
||||
,p.tld
|
||||
--,b.label as bias
|
||||
,pca.first
|
||||
,pca.second
|
||||
,s.cnt as stories
|
||||
FROM top.publisher_clusters_{source} c
|
||||
JOIN top.publishers p
|
||||
ON c.publisher_id = p.id
|
||||
JOIN
|
||||
(
|
||||
select
|
||||
s.publisher_id
|
||||
,count(1) as cnt
|
||||
FROM top.stories s
|
||||
GROUP BY
|
||||
s.publisher_id
|
||||
) s
|
||||
ON s.publisher_id = p.id
|
||||
JOIN top.publisher_pca_{source} pca
|
||||
ON pca.publisher_id = p.id
|
||||
""").df()
|
||||
DB.close()
|
||||
|
||||
ax = sns.scatterplot(x=df['first'], y=df['second'], hue=df['cluster'])
|
||||
ax.set(title=f"pca components vs. clusters ({source})", xlabel="first pca component", ylabel="second pca component")
|
||||
plt.savefig(out_dir / filename)
|
||||
|
||||
# .df().groupby(['cluster', 'bias']).describe()
|
||||
|
||||
|
||||
|
||||
|
||||
def test():
|
||||
data_dir = Path(os.getenv('DATA_MINING_DATA_DIR'))
|
||||
|
||||
DB.query("""
|
||||
SELECT
|
||||
p.id as publisher_id
|
||||
,p.name
|
||||
,p.tld
|
||||
,cast(b.bias_id as int) as bias_id
|
||||
,count(1) as stories
|
||||
FROM publishers p
|
||||
JOIN stories s
|
||||
ON s.publisher_id = p.id
|
||||
JOIN publisher_clusters c
|
||||
ON c.publisher_id = p.id
|
||||
LEFT JOIN publisher_bias b
|
||||
ON b.publisher_id = p.id
|
||||
where bias_id is null
|
||||
group by
|
||||
p.id
|
||||
,p.name
|
||||
,p.tld
|
||||
,b.bias_id
|
||||
ORDER BY count(1) desc
|
||||
""")
|
||||
|
||||
# .df().to_csv(data_dir / 'cluster_publishers.csv', sep="|", index=False)
|
||||
DB.close()
|
||||
|
|
@ -0,0 +1,48 @@
|
|||
from data.main import connect
|
||||
import pandas as pd
|
||||
import numpy as np
|
||||
|
||||
DB = connect()
|
||||
edges = DB.query("""
|
||||
select
|
||||
*
|
||||
from link_edges
|
||||
""").df()
|
||||
DB.close()
|
||||
|
||||
edges
|
||||
|
||||
adj = edges.pivot(index='parent_id', columns='child_id', values='links').fillna(0)
|
||||
select_publishers = pd.DataFrame(adj.index.tolist(), columns=['publisher_id'])
|
||||
|
||||
DB = connect()
|
||||
DB.query("create schema top")
|
||||
|
||||
DB.query("""
|
||||
CREATE OR REPLACE TABLE top.publishers AS
|
||||
SELECT
|
||||
p.*
|
||||
FROM publishers p
|
||||
JOIN select_publishers s
|
||||
ON s.publisher_id = p.id
|
||||
""")
|
||||
|
||||
DB.query("""
|
||||
CREATE OR REPLACE TABLE top.stories AS
|
||||
SELECT
|
||||
s.*
|
||||
FROM stories s
|
||||
JOIN top.publishers p
|
||||
ON s.publisher_id = p.id
|
||||
WHERE year(s.published_at) >= 2006
|
||||
AND year(s.published_at) < 2023
|
||||
""")
|
||||
|
||||
DB.query("""
|
||||
CREATE OR REPLACE TABLE top.related_stories AS
|
||||
SELECT
|
||||
r.*
|
||||
FROM top.stories s
|
||||
JOIN related_stories r
|
||||
ON s.id = r.parent_id
|
||||
""")
|
|
@ -0,0 +1,138 @@
|
|||
from transformers import AutoTokenizer, AutoModel
|
||||
import torch
|
||||
import torch.nn.functional as F
|
||||
from data.main import connect, data_dir
|
||||
import os
|
||||
from pathlib import Path
|
||||
import numpy as np
|
||||
import pandas as pd
|
||||
from tqdm import tqdm
|
||||
import click
|
||||
|
||||
#Mean Pooling - Take attention mask into account for correct averaging
|
||||
def mean_pooling(model_output, attention_mask):
|
||||
token_embeddings = model_output[0] #First element of model_output contains all token embeddings
|
||||
input_mask_expanded = attention_mask.unsqueeze(-1).expand(token_embeddings.size()).float()
|
||||
return torch.sum(token_embeddings * input_mask_expanded, 1) / torch.clamp(input_mask_expanded.sum(1), min=1e-9)
|
||||
|
||||
@click.option('-c', '--chunks', type=int, default=500, show_default=True)
|
||||
@click.command("sentence:embed")
|
||||
def embed(chunks):
|
||||
|
||||
# Load model from HuggingFace Hub
|
||||
tokenizer = AutoTokenizer.from_pretrained('sentence-transformers/all-MiniLM-L6-v2')
|
||||
model = AutoModel.from_pretrained('sentence-transformers/all-MiniLM-L6-v2')
|
||||
|
||||
# load data
|
||||
DB = connect()
|
||||
table = DB.sql("""
|
||||
select
|
||||
id
|
||||
,title
|
||||
from stories
|
||||
order by id desc
|
||||
""").df()
|
||||
DB.close()
|
||||
|
||||
# normalize text
|
||||
table['title'] = table['title'].str.normalize('NFKD').str.encode('ascii', errors='ignore').str.decode('utf-8')
|
||||
|
||||
|
||||
chunked = np.array_split(table, chunks)
|
||||
# generate embeddings from list of titles
|
||||
iterator = tqdm(chunked, 'embedding')
|
||||
embeddings = []
|
||||
embedding_ids = []
|
||||
for _, chunk in enumerate(iterator):
|
||||
sentences = chunk['title'].tolist()
|
||||
ids = chunk['id'].tolist()
|
||||
# Tokenize sentences
|
||||
encoded_input = tokenizer(sentences, padding=True, truncation=True, return_tensors='pt')
|
||||
# Compute token embeddings
|
||||
with torch.no_grad():
|
||||
model_output = model(**encoded_input)
|
||||
# Perform pooling
|
||||
output = mean_pooling(model_output, encoded_input['attention_mask'])
|
||||
# Normalize embeddings
|
||||
output = F.normalize(output, p=2, dim=1)
|
||||
embeddings.append(output)
|
||||
embedding_ids.append(ids)
|
||||
|
||||
embeddings = np.concatenate(embeddings)
|
||||
ids = np.concatenate(embedding_ids)
|
||||
|
||||
# save embeddings
|
||||
save_to = data_dir() / 'embeddings.npy'
|
||||
np.save(save_to, embeddings)
|
||||
print(f"embeddings saved: {save_to}")
|
||||
|
||||
# save ids
|
||||
save_to = data_dir() / 'embedding_ids.npy'
|
||||
np.save(save_to, ids)
|
||||
print(f"ids saved: {save_to}")
|
||||
|
||||
|
||||
@click.command('sentence:create-pca-table')
|
||||
def create_table():
|
||||
from sklearn import linear_model
|
||||
data_path = Path(os.getenv('DATA_MINING_DATA_DIR'))
|
||||
|
||||
embeddings = np.load(data_path / 'embeddings.npy')
|
||||
embedding_ids = np.load(data_path / 'embedding_ids.npy')
|
||||
ids = pd.DataFrame(embedding_ids, columns=['story_id']).reset_index()
|
||||
DB = connect()
|
||||
|
||||
data = DB.query("""
|
||||
SELECT
|
||||
ids.index
|
||||
,s.id
|
||||
,b.ordinal
|
||||
FROM ids
|
||||
JOIN top.stories s
|
||||
ON ids.story_id = s.id
|
||||
JOIN top.publisher_bias pb
|
||||
ON pb.publisher_id = s.publisher_id
|
||||
JOIN bias_ratings b
|
||||
ON b.id = pb.bias_id
|
||||
""").df()
|
||||
|
||||
x = embeddings[data['index']]
|
||||
y = data['ordinal'].to_numpy().reshape(-1, 1)
|
||||
|
||||
reg = linear_model.LinearRegression()
|
||||
|
||||
reg.fit(x, y)
|
||||
|
||||
reg.coef_.shape
|
||||
|
||||
@click.command('sentence:create-svm-table')
|
||||
def create_svm_table():
|
||||
from sklearn import svm
|
||||
data_path = Path(os.getenv('DATA_MINING_DATA_DIR'))
|
||||
|
||||
embeddings = np.load(data_path / 'embeddings.npy')
|
||||
embedding_ids = np.load(data_path / 'embedding_ids.npy')
|
||||
ids = pd.DataFrame(embedding_ids, columns=['story_id']).reset_index()
|
||||
|
||||
DB = connect()
|
||||
data = DB.query("""
|
||||
SELECT
|
||||
ids.index
|
||||
,s.id
|
||||
,b.ordinal
|
||||
FROM ids
|
||||
JOIN top.stories s
|
||||
ON ids.story_id = s.id
|
||||
JOIN top.publisher_bias pb
|
||||
ON pb.publisher_id = s.publisher_id
|
||||
JOIN bias_ratings b
|
||||
ON b.id = pb.bias_id
|
||||
""").df()
|
||||
|
||||
x = embeddings[data['index']]
|
||||
#y = data['ordinal'].to_numpy().reshape(-1, 1)
|
||||
y = data['ordinal']
|
||||
|
||||
clf = svm.SVC()
|
||||
pred = clf.fit(x, y)
|
||||
|
|
@ -0,0 +1,5 @@
|
|||
import train.main
|
||||
|
||||
__all__ = [
|
||||
'main'
|
||||
]
|
|
@ -0,0 +1,38 @@
|
|||
from torch.utils.data import Dataset
|
||||
from data.main import connect, data_dir
|
||||
from bias import label_to_int
|
||||
import numpy as np
|
||||
import pandas as pd
|
||||
|
||||
class NewsDataset(Dataset):
|
||||
def __init__(self):
|
||||
self.embeddings = np.load(data_dir() / 'embeddings.npy')
|
||||
embedding_ids = pd.DataFrame(np.load(data_dir() / 'embedding_ids.npy'), columns=['id']).reset_index()
|
||||
|
||||
DB = connect()
|
||||
query = """
|
||||
SELECT
|
||||
s.id
|
||||
,b.label
|
||||
,count(1) over (partition by publisher_id) as stories
|
||||
FROM stories s
|
||||
JOIN publisher_bias b
|
||||
ON b.id = s.publisher_id
|
||||
WHERE b.label != 'allsides'
|
||||
"""
|
||||
data = DB.sql(query).df()
|
||||
DB.close()
|
||||
|
||||
data['label'] = data['label'].apply(lambda x: label_to_int(x))
|
||||
data = data.merge(embedding_ids)
|
||||
self.data = data
|
||||
|
||||
def __len__(self):
|
||||
return len(self.data)
|
||||
|
||||
def __getitem__(self, idx):
|
||||
row = self.data.iloc[idx]
|
||||
y = row['label']
|
||||
# x = np.concatenate((self.embeddings[row['index']], [row['stories']])).astype(np.float32)
|
||||
x = self.embeddings[row['index']]
|
||||
return x, y
|
|
@ -0,0 +1,132 @@
|
|||
import click
|
||||
from tqdm import tqdm
|
||||
from enum import Enum, auto
|
||||
from dotenv import load_dotenv
|
||||
import os
|
||||
|
||||
import torch
|
||||
from torch import nn
|
||||
from torch import optim
|
||||
from torch.utils.data import DataLoader
|
||||
from accelerate import Accelerator
|
||||
|
||||
from train.dataset import NewsDataset
|
||||
from train.model import Classifier
|
||||
#from model.linear import LinearClassifier
|
||||
|
||||
class Stage(Enum):
|
||||
TRAIN = auto()
|
||||
DEV = auto()
|
||||
|
||||
@click.command('train:main')
|
||||
def main():
|
||||
dev_after = 20
|
||||
visible_devices = None
|
||||
lr = 1e-4
|
||||
epochs = 10
|
||||
debug = False
|
||||
torch.manual_seed(0)
|
||||
num_workers = 0
|
||||
|
||||
embedding_length = int(os.getenv('EMBEDDING_LENGTH', 384))
|
||||
|
||||
dataset = NewsDataset()
|
||||
trainset, devset = torch.utils.data.random_split(dataset, [0.8, 0.2])
|
||||
batch_size = 512
|
||||
trainloader = DataLoader(trainset, batch_size=batch_size, shuffle=True, num_workers=num_workers, drop_last=True)
|
||||
devloader = DataLoader(devset, shuffle=False, num_workers=num_workers)
|
||||
accelerator = Accelerator()
|
||||
model = Classifier(embedding_length=embedding_length, classes=5)
|
||||
|
||||
# it's possible to control which GPUs the process can see using an environmental variable
|
||||
if visible_devices:
|
||||
os.environ['CUDA_VISIBLE_DEVICES'] = visible_devices
|
||||
if debug:
|
||||
os.environ['CUDA_LAUNCH_BLOCKING'] = '1'
|
||||
#accelerator.log({"message" :"debug enabled"})
|
||||
|
||||
criterion = torch.nn.CrossEntropyLoss()
|
||||
optimizer = torch.optim.Adam(model.parameters(), lr=lr)
|
||||
|
||||
# wrap objects with accelerate
|
||||
model, optimizer, trainloader, devloader = accelerator.prepare(model, optimizer, trainloader, devloader)
|
||||
def run():
|
||||
"""runner for training and valdating"""
|
||||
running_loss = 0.0
|
||||
# set the model to train model
|
||||
model.train() if stage == Stage.TRAIN else model.eval()
|
||||
dataloader = trainloader if stage == Stage.TRAIN else devloader
|
||||
desc = 'train epoch' if stage == Stage.TRAIN else 'dev epoch'
|
||||
if debug:
|
||||
...
|
||||
|
||||
# Make sure there are no leftover gradients before starting training an epoch
|
||||
optimizer.zero_grad()
|
||||
|
||||
for batch, (x, y) in enumerate(tqdm(dataloader, desc=desc)):
|
||||
pred_y = model(x) # Forward pass through model
|
||||
loss = criterion(pred_y, y)
|
||||
running_loss += loss # Increment running loss
|
||||
# Only update model weights on training
|
||||
if stage == Stage.TRAIN:
|
||||
accelerator.backward(loss) # Increment gradients within model by sending loss backwards
|
||||
optimizer.step() # Update model weights
|
||||
optimizer.zero_grad() # Reset gradients to 0
|
||||
return running_loss / len(dataloader)
|
||||
|
||||
|
||||
for epoch in range(epochs):
|
||||
if (epoch - 1) % dev_after == 0:
|
||||
stage = Stage.DEV
|
||||
log = run()
|
||||
print(f"dev loss: {log}")
|
||||
else:
|
||||
stage = Stage.TRAIN
|
||||
log = run()
|
||||
print(f"train loss: {log}")
|
||||
|
||||
breakpoint()
|
||||
from data.main import data_dir, connect
|
||||
import numpy as np
|
||||
import pandas as pd
|
||||
from bias import int_to_label
|
||||
|
||||
embeddings = dataset.embeddings
|
||||
embedding_ids = dataset.data
|
||||
|
||||
DB = connect()
|
||||
query = """
|
||||
SELECT
|
||||
s.id
|
||||
,title
|
||||
,p.name
|
||||
,count(1) over (partition by publisher_id) as stories
|
||||
FROM stories s
|
||||
JOIN publishers p
|
||||
on p.id = s.publisher_id
|
||||
WHERE s.publisher_id NOT IN (
|
||||
SELECT
|
||||
id
|
||||
FROM publisher_bias b
|
||||
)
|
||||
"""
|
||||
data = DB.sql(query).df()
|
||||
embeddings = np.load(data_dir() / 'embeddings.npy')
|
||||
embedding_ids = pd.DataFrame(np.load(data_dir() / 'embedding_ids.npy'), columns=['id']).reset_index()
|
||||
|
||||
|
||||
for i in range(10):
|
||||
embedding = embeddings[embedding_ids[embedding_ids['id'] == data.iloc[i]['id']]['index']]
|
||||
title = data.iloc[i]['title']
|
||||
publisher = data.iloc[i]['name']
|
||||
class_pred = nn.functional.softmax( model(torch.tensor(embedding))).detach()
|
||||
class_id = int(torch.argmax(nn.functional.softmax( model(torch.tensor(embedding))).detach()))
|
||||
print(f"{publisher}: {int_to_label(class_id)} - \"{title}\"")
|
||||
|
||||
embedding_ids['id'] == data.iloc[0]['id']
|
||||
embedding_ids[embedding_ids['id'] == data.iloc[0]['id']]
|
||||
embedding = embeddings[embedding_ids[embedding_ids['id'] == data.iloc[0]['id']]['index']]
|
||||
title
|
||||
publisher
|
||||
|
||||
model().get_last_layer(torch.tensor(embedding))
|
|
@ -0,0 +1,28 @@
|
|||
from torch import nn
|
||||
|
||||
class Classifier(nn.Module):
|
||||
def __init__(self, embedding_length: int, classes: int):
|
||||
super().__init__()
|
||||
out_len = 16
|
||||
self.stack = nn.Sequential(
|
||||
nn.Linear(embedding_length, 256),
|
||||
nn.ReLU(),
|
||||
nn.Linear(256, 256),
|
||||
nn.ReLU(),
|
||||
nn.Linear(256, 64),
|
||||
nn.ReLU(),
|
||||
nn.Linear(64, 64),
|
||||
nn.ReLU(),
|
||||
nn.Linear(64, out_len),
|
||||
nn.ReLU(),
|
||||
)
|
||||
self.logits = nn.Linear(out_len, classes)
|
||||
|
||||
def forward(self, x):
|
||||
x = self.stack(x)
|
||||
self.last_hidden_layer = x.detach()
|
||||
return self.logits(x)
|
||||
|
||||
def get_last_layer(self, x):
|
||||
x = self.stack(x)
|
||||
return x
|
|
@ -1,7 +1,7 @@
|
|||
import click
|
||||
from transformers import AutoTokenizer, RobertaModel
|
||||
import numpy as np
|
||||
from data import Data, from_db, connect, data_dir
|
||||
from data.main import Data, from_db, connect, data_dir
|
||||
from tqdm import tqdm
|
||||
import torch
|
||||
from pathlib import Path
|
||||
|
|